A magazine where the digital world meets the real world.
On the web
- Home
- Browse by date
- Browse by topic
- Enter the maze
- Follow our blog
- Follow us on Twitter
- Resources for teachers
- Subscribe
In print
What is cs4fn?
- About us
- Contact us
- Partners
- Privacy and cookies
- Copyright and contributions
- Links to other fun sites
- Complete our questionnaire, give us feedback
Search:
Computers that read emotions
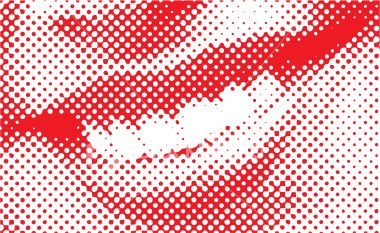
One of the ways that computers could be more like humans – and maybe pass the Turing test – is by responding to emotion. But how could a computer learn to read human emotions out of words? Matthew Purver of Queen Mary, University of London tells us how.
Have you ever thought about why you add emoticons to your text messages – symbols like :-) and :-@? Why do we do this with some messages but not with others? And why do we use different words, symbols and abbreviations in texts, Twitter messages, Facebook status updates and formal writing?
In face-to-face conversation, we get a lot of information from the way someone sounds, their facial expressions, and their gestures. In particular, this is the way we convey much of our emotional information – how happy or annoyed we're feeling about what we're saying. But when we're sending a written message, these audio-visual cues are lost – so we have to think of other ways to convey the same information. The ways we choose to do this depend on the space we have available, and on what we think other people will understand. If we're writing a book or an article, with lots of space and time available, we can use extra words to fully describe our point of view. But if we're writing an SMS message when we're short of time and the phone keypad takes time to use, or if we're writing on Twitter and only have 140 characters of space, then we need to think of other conventions. Humans are very good at this – we can invent and understand new symbols, words or abbreviations quite easily. If you hadn't seen the :-D symbol before, you can probably guess what it means – especially if you know something about the person texting you, and what you're talking about.
But computers are terrible at this. They're generally bad at guessing new things, and they're bad at understanding the way we naturally express ourselves. So if computers need to understand what people are writing to each other in short messages like on Twitter or Facebook, we have a problem. But this is something researchers would really like to do: for example, researchers in France, Germany and Ireland have all found that Twitter opinions can help predict election results, sometimes better than standard exit polls – and if we could accurately understand whether people are feeling happy or angry about a candidate when they tweet about them, we'd have a powerful tool for understanding popular opinion. Similarly we could automatically find out whether people liked a new product when it was launched; and some research even suggests you could even predict the stock market. But how do we teach computers to understand emotional content, and learn to adapt to the new ways we express it?
One answer might be in a class of techniques called semi-supervised learning. By taking some example messages in which the authors have made the emotional content very clear (using emoticons, or specific conventions like Twitter's #fail or abbreviations like LOL), we can give ourselves a foundation to build on. A computer can learn the words and phrases that seem to be associated with these clear emotions, so it understands this limited set of messages. Then, by allowing it to find new data with the same words and phrases, it can learn new examples for itself. Eventually, it can learn new symbols or phrases if it sees them together with emotional patterns it already knows enough times to be confident, and then we're on our way towards an emotionally aware computer. However, we're still a fair way off getting it right all the time, every time.