A magazine where the digital world meets the real world.
On the web
- Home
- Browse by date
- Browse by topic
- Enter the maze
- Follow our blog
- Follow us on Twitter
- Resources for teachers
- Subscribe
In print
What is cs4fn?
- About us
- Contact us
- Partners
- Privacy and cookies
- Copyright and contributions
- Links to other fun sites
- Complete our questionnaire, give us feedback
Search:
Software for justice
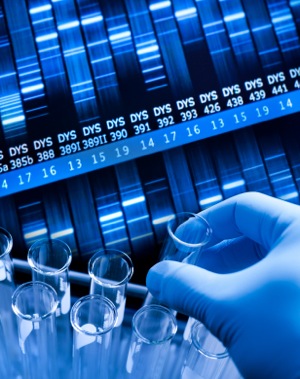
A jury is given misleading information in court by an expert witness. An innocent person goes to prison as a result. This shouldn't happen, but unfortunately it does and more often than you might hope. It's not because the experts or lawyers are trying to mislead but because of some tricky mathematics. Fortunately, a team of computer scientists at Queen Mary, University of London are leading the way in fixing the problem.
The Queen Mary team, led by Professor Norman Fenton, is trying to ensure that forensic evidence involving probability and statistics can be presented without making errors, even when the evidence is incredibly complex. Their solution is based on specialist software they have developed.
Many cases in courts rely on evidence like DNA and fibre matching for proof. When police investigators find traces of this kind of evidence from the crime scene they try to link it to a suspect. But there is a lot of misunderstanding about what it means to find a match. Surprisingly, a DNA match between, say, a trace of blood found at the scene and blood taken from a suspect does not mean that the trace must have come from the suspect.
Forensic experts talk about a 'random match probability'. It is just the probability that the suspect's DNA matches the trace if it did not actually come from him or her. Even a one-in-a-billion random match probability does not prove it was the suspect's trace. Worse, the random match probability an expert witness might give is often either wrong or misleading. This can be because it fails to take account of potential cross-contamination, which happens when samples of evidence accidentally get mixed together, or even when officers leave traces of their own DNA from handling the evidence. It can also be wrong due to mistakes in the way the evidence was collected or tested. Other problems arise if family members aren't explicitly ruled out, as that makes the random match probability much higher. When the forensic match is from fibre or glass, the random match probabilities are even more uncertain.
The potential to get the probabilities wrong isn't restricted to errors in the match statistics, either. Suppose the match probability is one in ten thousand. When the experts or lawyers present this evidence they often say things like: "The probability that the trace came from anybody other than the defendant is one in ten thousand." That statement sounds OK but it isn't true.
The problem is called the prosecutor fallacy. You can't actually conclude anything about the probability that the trace belonged to the defendant unless you know something about the number of potential suspects. Suppose this is the only evidence against the defendant and that the crime happened on an island where the defendant was one of a million adults who could have committed the crime. Then the random match probability of one in ten thousand actually means that about one hundred of those million adults match the trace. So the probability of innocence is ninety-nine out of a hundred! That's very different from the one in ten thousand probability implied by the statement given in court.
Norman Fenton's work is based around a theorem, called Bayes' theorem, which gives the correct way to calculate these kinds of probabilities. The theorem is over 250 years old but it is widely misunderstood and, in all but the simplest cases is very difficult to calculate properly. Most cases include many pieces of related evidence – including evidence about the accuracy of the testing processes. To keep everything straight, experts need to build a model called a Bayesian network. It’s like a graph that maps out different possibilities and the chances that they are true. You can imagine that in almost any court case, this gets complicated awfully quickly. It is only in the last 20 years that researchers have discovered ways to perform the calculations for Bayesian networks, and written software to help them. What Norman and his team have done is develop methods specifically for modelling legal evidence as Bayesian networks in ways that are understandable by lawyers and expert witnesses.
Norman and his colleague Martin Neil have provided expert evidence (for lawyers) using these methods in several high-profile cases. Their methods help lawyers to determine the true value of any piece of evidence – individually or in combination. They also help show how to present probabilistic arguments properly.
Unfortunately, although scientists accept that Bayes' theorem is the only viable method for reasoning about probabilistic evidence, it's not often used in court, and is even a little controversial. Norman is leading an international group to help bring Bayes' theorem a little more love from lawyers, judges and forensic scientists. Although changes in legal practice happen very slowly (lawyers still wear powdered wigs, after all), hopefully in the future the difficult job of judging evidence will be made easier and fairer with the help of Bayes' theorem.
If that happens, then thanks to some 250 year-old maths combined with some very modern computer science, fewer innocent people will end up in jail. Given the innocent person in the dock could one day be you, you will probably agree that's a good thing.