A magazine where the digital world meets the real world.
On the web
- Home
- Browse by date
- Browse by topic
- Enter the maze
- Follow our blog
- Follow us on Twitter
- Resources for teachers
- Subscribe
In print
What is cs4fn?
- About us
- Contact us
- Partners
- Privacy and cookies
- Copyright and contributions
- Links to other fun sites
- Complete our questionnaire, give us feedback
Search:
Predicting Cancer Cures
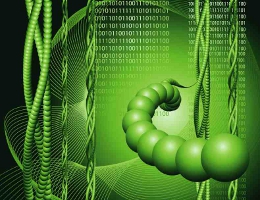
The most exciting research often comes when people from different subjects work together, and it can come from unexpected directions. How might Computer Scientists help in the discovery of drugs to fight Cancer? The obvious way is in building faster super-computers to number-crunch the problems. However, there can be much more subtle ways ideas from one area can have a big impact on others if the researchers are creative enough to see the links. Prof. Muffy Calder, a Computer Scientist at Glasgow University, discovered one intriguing link while working with cancer specialists. It turns out that the problems of understanding how drugs act on our cell chemistry are very similar to those in understanding communication networks. Tools for one can be used for the other.
Muffy and team are aiming to understand the biochemical "pathways" in which signals pass through from the membrane of cells into their nucleus. These "pathways" are just a series of chemical reactions where different protein molecules are created and destroyed. To develop cancer drugs scientists need to have a better understanding of how this happens. It will help them predict the way the reactions are affected by drugs.
The pathways are normally modelled using complex maths built into tools that simulate the processes involved - allowing virtual experiments to be done on the computer instead of on real cells. These simulations are used to suggest actual experiments and to help understand the results. Muffy's team realised that the diagrams used by biochemists to illustrate the reactions are well known to computer scientists - they look just like the "producer-consumer" networks already used to analyse telecom networks. That means tools already developed for analysing telecom networks can be used to analyse the biological networks. What advantage does this give over the use of the maths? Proof can be used rather than just simulation. Simulation allows you to check what happens in particular individual situations: the ones you simulate. Unfortunately other situations may or may not give the same results: you don't know unless you simulate them too. With the proof-based tools, general properties of the biological system as modelled can be shown to always be true. You can, for example, work out the probability that too much of a particular protein will be produced.
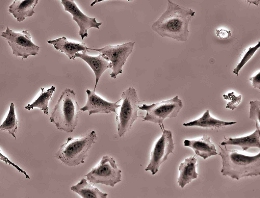
Previous work like this had a focus on proving properties at a molecular level: what will happen if single molecules react? Muffy realised that when developing drugs it's not what single molecules do that ultimately matters, but being able to predict how test tubes of reagents behave. That means you have to model something slightly different: what's known as the molar level, the test-tube level. It turns out that to prove properties interesting to the biochemists only two levels of concentration of reagents matter - high and low - corresponding to enough and not enough reagent to trigger a reaction. This is a similar idea to one computer scientists use to reason about hardware circuits, thinking in terms of high and low voltage levels rather than about individual electrons.
The work opens up a whole new approach for developing drugs. The ultimate aim is to provide predictive tools for biochemists. They will suggest what effects different drugs might have on the processes taking place in cells and so suggest experiments to perform. If the team of computer scientists and cancer specialists do manage this, they will have handed the biochemists a powerful new tool to help in the fight against cancer.